Keynote Speakers
Prof. Ko Nishino (Drexel University) [webpage]
Title: Going with the flow: modeling and exploiting crowd flow in videos
Abstract:
Computer vision research, in the past few decades, has made large strides toward efficient and reliable processing
of the ever increasing video data, especially for surveillance purposes.
Automated visual analysis of crowded scenes, however, remains a challenging task.
As the number of people in a scene increases, nuisances that play against conventional video analysis methods surge.
People will occlude each other, the notion of foreground and background collapses,
and most important the behavior of the scene content especially of those of people will change to accommodate the clutter in the scene.
These are nuisances not only to the computer algorithms but also to human operators that will have to squint through the clutter
for hours and days to find a single adverse activity.
In other words, automated video analysis is most needed in crowded scenes where it is hardest to do.
The crowd, however, does in turn give rise to invaluable visual cues regarding the scene dynamics. The appearance of a large number of people densely packed in the scene adds texture to the emerging movement of the people as a group--the crowd flow. If we can model the crowd flow while faithfully encoding their variability both in space and time, we may use it to extract important information about the dynamic scene. In this talk, I will discuss about learning a statistical model of the spatially and temporally varying local motion patterns underlying the crowd flow and show how we can use it to achieve challenging video analysis tasks, in particular anomaly detection and pedestrian tracking, in extremely crowded scenes.
The crowd, however, does in turn give rise to invaluable visual cues regarding the scene dynamics. The appearance of a large number of people densely packed in the scene adds texture to the emerging movement of the people as a group--the crowd flow. If we can model the crowd flow while faithfully encoding their variability both in space and time, we may use it to extract important information about the dynamic scene. In this talk, I will discuss about learning a statistical model of the spatially and temporally varying local motion patterns underlying the crowd flow and show how we can use it to achieve challenging video analysis tasks, in particular anomaly detection and pedestrian tracking, in extremely crowded scenes.
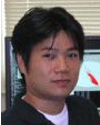
Ko Nishino received BE and ME degrees in Information and Communication Engineering and
a PhD degree in Computer Science from the University of Tokyo in 1997, 1999, and 2002, respectively.
He joined the Department of Computer Science at Drexel University as an Assistant Professor in 2005 and was promoted to Associate Professor in 2011.
He currently serves as the Director of Graduate Affairs and Research of the Department of Computing in the newly formed College of Computing and Informatics.
Prior to joining Drexel University, he was a Postdoctoral Research Scientist in the Department of Computer Science at Columbia University.
His research interests mainly lie in computer vision and its intersections with computer graphics, machine learning, and digital archaeology.
The main focus of his research is on photometric and geometric modeling of real-world objects and scenes.
He has published a number of papers on related topics, including physics-based vision,
image-based modeling and rendering, geometry processing, and video analysis.
He received the NSF CAREER Award in 2008.
He is a member of the IEEE and the ACM.
Dr. Alireza Fathi (Stanford University) [webpage]
Title: Learning to Recognize Objects and Activities in Egocentric Video
Abstract:
Recent advances in camera technology have made it possible to build a comfortable, wearable system
which can capture the scene in front of the user as they go about their daily life.
Products based on this technology, such as GoPro and Google Glass, have generated substantial interest.
In this talk, I will present my work on egocentric vision, which leverages wearable camera technology and
provides a new line of attack on classical computer vision problems such as object categorization and activity recognition.
I will demonstrate that contextual cues and the actions of a user can be exploited in an egocentric vision system
to learn models of objects under very weak supervision.
In addition, I will show that measurements of a subject’s gaze during object manipulation tasks can provide
novel feature representations to support activity recognition.
Moving beyond surface-level categorization, I will showcase a method for automatically discovering object state changes during actions,
and an approach to building descriptive models of social interactions between groups of individuals.
These new capabilities for egocentric video analysis will enable new applications in life logging, elder care,
human-robot interaction, developmental screening, augmented reality and social media.
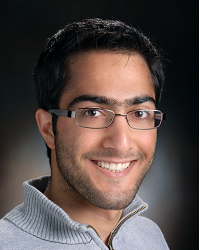
Alireza Fathi received his Ph.D. from Georgia Institute of Technology in 2013,
working with James M. Rehg and joined FeiFei Li's lab at Stanford University
as a postdoctoral fellow in July 2013.
He received a MSc degrees from Georgia Tech in 2013 and
one from Simon Fraser University in Canada in 2008.
He received his Bachelor’s degree from Sharif University of Technology in Iran in 2006.
His main research areas are computer vision and machine learning, with a particular interest in egocentric (first-person) vision.
He has published several papers at the top vision conferences, including CVPR, ICCV and ECCV,
on recognizing objects and activities in first-person view videos.
He was a co-organizer of the 2nd IEEE Workshop on Egocentric Vision,
which was held in conjunction with CVPR 2012.
Prof. Hanako Yoshida (University of Houston) [webpage]
Title: The role of parent and infant hand actions in creating critical information for the developing visual system
Abstract:
Children learn about their world through social interactions, whether it be about objects, actions, or other social beings.
What children attend to in these events is generated partly by their own actions
- directed eye gaze, head movements, posture shifts, objects grabbed/held - and by the same actions performed by the social partner.
We seek to understand the dynamic structure of embodied attention in the context of social learning (e.g., toy play with a parent labeling toys).
I will discuss a method for exploring the dynamics of early attention from the child’s point of view. With this study,
we place a small head camera/eye tracking device on the child as the child is engaged in a social learning context (e.g., toy play with a parent labeling toys)
to capture events from the child’s perspective.
We will review some previous attempts observing such child centered views with toddlers,
and introduce the most recent longitudinal study using head-mounted eye tracking system
which explores such interactions in 10 developing children every 3 months from the age of 6 months to 18 months.
If time allows, I will be also prepared to introduce one of our unique applications of this head-mounted eye tracking system,
which enables us to study children's attentional strategies during a laboratory task engagement.
The findings will be discussed in relation to the nature of early input, and how individuals' bodily experiences interact with learning.
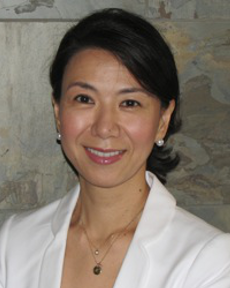
Hanako Yoshida is an associate professor in the Department of Psychology at University of Houston.
She received a B.A. and a Ph.D. in psychology in 1998 and 2003, respectively, from Indiana University under the supervision of Dr. Linda B. Smith.
Before joining University of Houston in 2006, she was a Postdoctoral Research Scientist in the Psychological and Brain Sciences Program at Indiana University.
Her primary research concerns how contextual cues (e.g., words, perceptual cues, and social cues) organize infants’ and children’s attention, getting it exquisitely tuned to immediate task demands and further propelling future learning.
She has a demonstrated record of successful and productive research programs and has published her work in respected journals including Cognition, Developmental Science, Psychological Sciences, and Infancy.