Program
This workshop is organized by two research groups supported by JST-CREST. Two keynote speakers are leaders of the groups and their colaborators give talks about the newest achievement of these research projects.
Tentative program:
Keynote Talks
[Keynote1] Prof. Yasushi Yagi (Osaka Univ.)
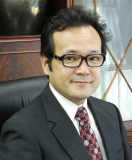
Title: Gait Video Analysis and Its Applications
Abstract: We have been studying human gait analysis for more than ten years. Considering the fact that everyone's walking style is unique, a human gait can be applied for person authentication task. Fortunately, our gait analysis technologies are getting to be used in real criminal investigation. We have constructed a large-scale gait database, and proposed several methods of gait analysis. The appearance of gait patterns are influenced by the changes of viewpoints, walking directions, speeds, clothes, and shoes. To overcome this problems, we have proposed several approaches with a part-based method, an appearance-based view transformation model, a periodic temporal super resolution method, a manifold based method and score level fusion. We show the efficiency of our approaches by evaluating on our large gait database. Furthermore, I introduce a novel research project "Behavior Understanding based on Intention-Gait Model" supported by JST-CREST in 2010. In this project, we focus on a new aspect that a human gait pattern is influenced by one's emotion, the object of one's activity, one's physical/mental conditions, and surrounding people. In this talk, I briefly introduce overview of this project and some studies on medical application.
[Keynote2] Dr. Takayuki Kanda (ATR)
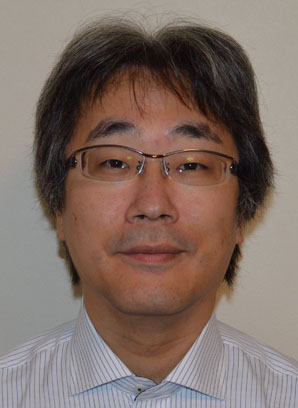
Title: Enabling a Mobile Social Robot to Adapt to a Public Space
Abstract: Robots are seemingly coming to appear in our daily lives. Yet, it is not as easy as one might imagine. In this talk, I plan to introduce the difficulties we faced and challenged with during we deploy robots to daily environments, such as shopping malls. It includes technical challenge as well as challenges in human-robot interaction (HRI).
Invited Talks
Chengju Zhou (Osaka Univ.)
Title: Detection of Gait Impairment in the Elderly Using Patch-GEI
Abstract: We propose a novel method for estimating physical impairment of elderly people using gait. To achieve this, we first investigate which gait feature is effective for this purpose among gait energy image (GEI), duration time, and phase fluctuation as dynamic features. GEI is a popular appearance-based feature showing high performance in human authentication. By comparison, we find that it is the most reasonable feature. In real situations, however, GEI is easily affected by clothes variations or carrying conditions, so that the use of whole body results in decreasing performance. Considering this problem, we thus propose to use only the GEI features of the most discriminative body patches. From the experiments that evaluate the contribution of various sizes of body patches, we find that head and chest regions perform better than the whole body with the classification accuracy improved from 80.93% to 83.17% for the visual impairment discrimination case. As for the leg impairment detection case, the leg region performs better than the whole body by an accuracy increased from 69.30% to 75.05%. These results confirm the effectiveness of patch-GEI for impairment detection.
Masataka Niwa (Osaka Univ.)
Title: Estimating the Elderly People’s Cognitive Functions from the Dual Task Gait
Abstract: In recent years, the numbers of elderly people have been increasing. We aim to evaluate elderly people's walking ability, physical function and cognitive function from their gait of walking or stepping in place. For this purpose, it is necessary to measure the large number of the elderly people's gait in the nursing home or elderly facility. Then, we proposed a measuring system for the elderly people who need nursing care or nursing support. We measured elderly people's gait in normal walking using this system. And, we analyzed these gait data. This analysis showed that physical functions will be able to be evaluated from the gait of elderly people. However, cognitive functions were not able to be evaluated from their gait in normal walking. Then, we measured elderly people's gait in walking with cognitive load (dual-task). To compare with this result, we gave elderly people the examination of dementia: Mini Mental State Examination (MMSE). It was suggested as the result of analysis that cognitive function will be able to estimate from the number of answer, the dispersion of answer interval and stability of stepping or answering correlate in dual task condition.
Fumio Okura (Osaka Univ.)
Title: Automatically Acquiring Walking-Related Behavior of 100,000 People
Abstract:
Acquiring large population human behavior under a controlled environment is an important yet a difficult problem. Using surveillance cameras installed on public spaces is a relatively easy way to construct large database; while it is difficult to control the specific behavior such as walking direction. Another strategy is to hire participants so that we can ask to do specific tasks. However, the population is limited according to the budget and workload; for example, one of the world largest dataset for walking behavior (The OU-ISIR Gait Database [Iwama, et al. 2012]) includes approximately 4,000 subjects.
We aim to construct two large population datasets that consist of 100,000 subjects. The datasets include human walking-related behaviors: 1) walking and 2) stepping during calculation. To observe walking behavior, we developed a capturing system using multiple cameras and photocells for automatic operation. The other system that employs an RGB-D camera and a pressure sensor captures a dual task of stepping and calculation, which is used for analyzing the cognitive ability through the human behavior. While acquiring the behavior of the participants, the systems display information attracting their interest such as the participants’ walking characteristics and the scores of the cognitive level. These systems currently operate in a science museum for nine months from July 2015; while >30,000 participants have already contributed in the first three months.
Hitoshi Habe (Kinki Univ.)
Title: Relevant Feature Extraction for Social Group Segmentation in the Real World
Abstract: We propose a method for estimating group membership in public spaces such as station and shopping mall. Social group estimation is an important step toward a deeper understanding of human behavior. For example, group attributes and its purpose are useful information to provide appropriate assistance for them. Our method utilizes pedestrian trajectory and their chest orientations captured by range sensors and extracts features which reflects the social relationship between two persons from. One challenge we have is it is difficult to obtain solely relevant features for group segmentation. Even when we are walking with a friend or colleague, we do not interact with the others all of the time. This means that a meaningful information for group detection is embedded within a part of time-series data, not all of the data. For picking up the relevant features and ignore the others we make use of multiple instance learning (MIL). MIL can extract a relevant feature included in a bag even when the bag also has other irrelevant features. We conduct experiments using captured data based on realistic scenarios The results demonstrate our method outperform an existing method.
Drazen Brscic (ATR)
Title: Open Datasets of Pedestrian Activity in Public Spaces
Abstract: Datasets serve as an important element for the advancement of research. They provide both a testbed for trying out novel algorithms as well as a benchmark for comparing the results to state-of-the art algorithms. In comparison to topics such as person recognition using computer vision, there has been a relative lack of datasets containing the pedestrian activity taken in real world environments. This is mainly due to the difficulty of collecting people's data in large public spaces and for extended periods of time. In this work we introduce a number of datasets that we made open to the public. The first one is a collection of trajectories of people passing through a large area of a shopping mall. This data was collected twice per week over a one-year period. The second dataset introduces manually annotated groups in both part of the first dataset as well as in a different shopping mall. Finally, the third dataset contains the trajectories of people approaching a social robot to interact.
Drazen Brscic (ATR)
Title: Detecting Anomalous Pedestrian Behavior from Trajectories
Abstract: We report a technique to detect pedestrians who walk in an anomalous way (e.g. people who got lost, suspicious people, etc.). Our approach is to prepare a motion model of normal pedestrians from a large amount of pedestrian trajectories, and then compute whether each trajectory is predictable from the pedestrian motion model. Our technique first decomposes trajectories into directions of major movements, from which sub-goals (locations toward which people walk) and probabilities of transitions between sub-goals are computed. The set of sub-goals and transitions enables us to predict a near-future behavior of each pedestrian. Hence, predictability of a pedestrian's trajectory can be computed as a deviation of the current location from the location predicted based on an earlier part of the trajectory. Finally, anomalous behavior is classified using the predictability. We manually labeled anomalous pedestrian behaviors in a large set of pedestrian trajectories collected in a real shopping mall, and obtained a dataset of typical-anomalous trajectories. We trained our model and evaluated on this dataset. Our method successfully classified 91.4% of trajectories, which outperformed alternative methods.
Satoru Satake (ATR)
Title: How to Build an Service Robot in Public Space: Extracting Attributes of Pedestrians for Human-Robot Interaction
Abstract: One expectation of a social robot is services on public space. But to achieve such services , the robot should use attributes of pedestrians for human-robot interactions. This talk shows what kind of attributes are necessary and useful for the services, and strategy to how to use it.
Deneth Karunarathne (ATR)
Title: A Communication Robot Walking Side-by-Side in a Real Environment
Abstract: We present a side-by-side walking robot working in a real environment where the final destination is unknown to the robot. This is an extension of a previous study where we only considered side-by-side walking with unknown destination in an indoor constrained experimental environment with few motion options. In contrast, this study presents an improved side-by-side model tested in a much more realistic and dynamic environment. In order to construct the model we first recorded a number of trajectories of people walking side-by-side in the same environment. The model contains a number of parameters we defined by observing the human side-by-side walking, which we calibrated based on the recorded trajectories. Finally, we evaluated the following. First, that the proposed model considering mutual utilities and sub-goals, and not considering the final destination performs as effectively and robustly as a model where the destination is known. Next we evaluated that the use of sub-goals is much efficient than not using of sub-goals. Finally the robot was deployed in the same environment where we recorded the earlier trajectories and proved to be effective in doing window-shopping with the participants.